FRAMEWORK BASED ON FUZZY EXPERT SYSTEM MODEL FOR PREDICTION OF ELECTRIC LOAD DEMAND-SUPPLY BALANCE
Keywords:
post privatization, load prediction, Framework, Fuzzy Expert SystemAbstract
Fuzzy expert system model is a resilience approach in short term predicting of electricity load. In this study, the post privatisation electricity framework in Nigeria was examined and found that, load distribution was not based on the demand by the users which often led to underutilization of load transmitted to the distribution company despite the fact that, the available power is not sufficient. The contribution to the framework is to enable distribution of electricity that is based on location specific load requirement. To achieve this, fuzzy expert system is integrated to the post privatisation framework with temperature, humidity, rainstorm, time of the day, previous load history, standard of living and history of previous bill payment were used as the inputs parameter for the fuzzy expert system. The integration of the fuzzy expert model to the previous framework is to predict electricity that is affordable to the users thereby reducing the amount of the unused electricity as well as reducing the accumulated bill resulted from the inability of the users to pay for what it consume.
Downloads
References
Advisory Power Team, Office of the Vice President, F. G. of N., & Power Africa. (2015). Nigeria Power Baseline Report. In Federal Government of Nigeria. Retrieved from https://mypower.ng/wp-content/uploads/2018/01/Baseline-Report.pdf
Onochie, U. P., Egware, H. O., & Eyakwanor, T. O. (2015). The Nigeria Electric Power Sector ( Opportunities and Challenges ). 2(4).
Usman, H., & Polytechnic, K. (2010). MAJOR FACTORS AFFECTING ELECTRICITY GENERATION, TRANSMISSION AND DISTRIBUTION IN NIGERIA Sule, A. H. 1, 159–164.
Konica, J. A., & Hanelli, L. (2016). Forecasting Next-Day the Electricity Demand Based On Fuzzy Logic Method Case for Albania. Management, 3(12), 6172–6180. Retrieved from https://pdfs.semanticscholar.org/f355/4ed50a3694dfd161215ccf54dbbecf376c4f.pdf
Cecati, C., Kolbusz, J., Rózycki, P., Siano, P., & Wilamowski, B. M. (2015). A Novel RBF Training Algorithm for Short-Term Electric Load Forecasting and Comparative Studies. IEEE Transactions on Industrial Electronics, 62(10), 6519–6529.
Manlook R., Badran O. & Abdulhadi E. (2015) A Fuzzy Inference Model for Short-term Load Forecasting. Energy Policy, Vol. 37 issue 3
Ertugrul, Ö. F. (2016). Forecasting electricity load by a novel recurrent extreme learning machines approach. International Journal of Electrical Power and Energy Systems, 78, 429–435. https://doi.org/10.1016/j.ijepes.2015.12.006.
Le, C. (2016). Type-2 Fuzzy Logic System Applications for Power Systems. (December). Anwana, E. O. (2016). Power Sector Reforms and Electricity Supply Growth in. 3(1), 94–102. https://doi.org/10.20448/journal.501/2016.3.1/501.1.94.102
Khosravanian, R., Sabah, M., Wood, D. A., & Shahryari, A. (2016). Journal of Natural Gas Science and Engineering Weight on drill bit prediction models : Sugeno-type and Mamdani-type fuzzy inference systems compared. Journal of Natural Gas Science
and Engineering, 36, 280–297. https://doi.org/10.1016/j.jngse.2016.10.046
Ali, D., Yohanna, M., Ijasini, P. M., & Garkida, M. B. (2018). Application of fuzzy – Neuro to model weather parameter variability impacts on electrical load based on long-term forecasting. Alexandria Engineering Journal, 57(1), 223–233. https://doi.org/10.1016/j.aej.2016.12.008
Ali, D., Yohanna, M., Puwu, M. I., & Garkida, B. M. (2016). Long-term load forecast modelling using a fuzzy logic approach. Pacific Science Review A: Natural Science and Engineering, 18(2), 123–127. https://doi.org/10.1016/j.psra.2016.09.011
Singh, M., & Kaur, G. (2019). Electric Load and Solar Irradiance Forecasting in Microgrid using High Order MIMO Fuzzy Logic Approach. International Journal of Advanced Engineering Research and Science, 6495(4).
Ozsahin, D. U., Gökçekuş, H., Uzun, B., & James, L. (2021). Application of Multi-Criteria Decision Analysis in Environmental and Civil Engineering. In Book: https://doi.org/10.1007/978-3-030-64765-0
Benson, S. A., & Ogunjuyigbe, J. K. (2018). Impact of weather variables on electricity power demand forecast using fuzzy logic technique. Nigerian Journal of Technology, 37(2), 450. https://doi.org/10.4314/njt.v37i2.21
Islas, M. A., Rubio, J. de J., Muñiz, S., Ochoa, G., Pacheco, J., Meda-Campaña, J. A., … Zacarias, A. (2021). A fuzzy logic model for hourly electrical power demand modeling. Electronics (Switzerland), 10(4), 1–12. https://doi.org/10.3390/electronics10040448
Ahmad, W., Ayub, N., Ali, T., Irfan, M., Awais, M., Shiraz, M., & Glowacz, A. (2020). Towards short term electricity load forecasting using improved support vector machine and extreme learning machine. Energies, 13(11), 1–17. https://doi.org/10.3390/en13112907
Tudose, A. M., Picioroaga, I. I., Sidea, D. O., Bulac, C., & Boicea, V. A. (2021). Short-term load forecasting using convolutional neural networks in covid-19 context: The Romanian case study. Energies, 14(13). https://doi.org/10.3390/en14134046
Singla M. K. & Hans, S. (2018) Load Forecasting using Fuzzy Logic Tool Box. Global Research and Development Journal for Engineering volume 8 issue 3
Madrid, E. A., & Antonio, N. (2021). Short-term electricity load forecasting with machine learning. Information (Switzerland), 12(2), 1–21. https://doi.org/10.3390/info12020050
Panda, S. K., Ray, P., & Mishra, D. P. (2021). Short Term Load Forecasting using Metaheuristic Techniques. IOP Conference Series: Materials Science and Engineering, 1033(1). https://doi.org/10.1088/1757-899X/1033/1/012016
Tripathi, S. P., Shukla, P. K., & Poonam. (2012). Uncertainty Handling using Fuzzy Logic in Rule Based Systems. International Journal of Advanced Science and Technology, 45, 31–46.
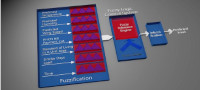
Downloads
Published
Issue
Section
License
Copyright (c) 2024 The Transactions of the Nigerian Association of Mathematical Physics

This work is licensed under a Creative Commons Attribution-NonCommercial-ShareAlike 4.0 International License.