A SYSTEM FOR THE DETECTION OF MALICIOUS DOMAIN NAMES USING IMPROVED DEEP-LEARNING MODEL
DOI:
https://doi.org/10.60787/tnamp-19-63-70Keywords:
Malicious Domain Detection, DNS, Cyber SecurityAbstract
The tremendous growth of innovative technologies used for online services in the global economic space brings vulnerabilities to security breaches. The upsurge of these vulnerabilities created a level playing field for cyber-attacks to flourish, with assailants constantly adapting new nefarious methods to compromise information and deceive naïve users of the cyberspace. Despite the amazing and numerous anti-phishing approaches and solutions, the increasing incidences caused by malicious domain name system attacks such as spam, phishing and malware could be attributed to the dynamism in the approaches used by cyber criminals to counterfeit the techniques. To address these issues, many cyber security researchers have switched their focus to machine learning-based methodologies for malicious DNS detection. In this paper, we introduced the usage of machine-based model to detect the dynamism of malicious DNS by exploring Machine Learning, Ensemble learning and Deep-Learning. A customized web Crawler was implemented to extract URL attribute for model extraction. Furthermore, a Cross validation approach was used towards the classification and regression metrics (statistical approach) to evaluate their performance to an accuracy of 89.9%. Our experiment is based on both active and passive DNS analysis.
Downloads
References
Li, K., Yu, X., & Wang, J. (2021) A Review: How to Detect Malicious Domains. In Advances in Artificial Intelligence and Security: 7th International Conference, ICAIS Dublin, Ireland, July 19-23, 2021, Proceedings, Part III 7 (pp. 152-162). (2021) Springer International Publishing.
Hamroun, C., Amamou, A., Haddadou, K., Haroun, H., &Pujolle, G.(2022.) A review on lexical based MDN detection methods. 6th Cyber Security in Networking Conference (CSNet) (pp. 1-7). IEEE.
Egwali A. O. and Alile S. O. (2020). A Casual Network Based System For Predicting Multi-Stage Attack with Malicious IP. International Journal of Academic Multidisciplinary Research 4 (5), 1-8.
Alile S. O. and Egwali A. O. (2020). A Bayesian Belief Network Model For Detecting Multi-stage Attacks With Malicious IP Addresses. I.J. Wireless and Microwave Technologies, 2, 30-41
Bilge L., Kirda, Kruegel E., Balduzzi C. (2011). EXPOSURE. Finding Malicious Domains Using Passive DNS Analysis. In Proceedings of the 18th Network and Distributed System Security Symposium, San Diego, CA, USA, 6 February 2011; Internet Society: Reston, VA, USA, PP. 1-17.
Hong Zhao, Zhaobin Chang, GuangbinBao, and Xiangyan Zeng.(2019). MDNs detection algorithm based on N-Gram. Journal of Computer Networks and Communications, Volume 2019, Article ID 4612474, page 9 Publish 3 February 2019. Guest Editor: Saman S. Chaeikar.
Halgamuge, M. N. (2022). Estimation of the success probability of a malicious attacker on blockchain-based edge network. Computer Networks, 219, 109402.
Ren, F., Jiang, Z., Wang, X., & Liu, J. (2020). A DGA domain names detection modeling method based on integrating an attention mechanism and deep neural network. Cybersecurity, 3(1), 1-13.
Ayub, M. A., Smith, S., Siraj, A., & Tinker, P. (2021, June). Domain Generating Algorithm based Malicious Domains Detection. In 2021 8th IEEE International Conference on Cyber Security and Cloud Computing (CSCloud)/2021 7th IEEE International Conference on Edge Computing and Scalable Cloud (EdgeCom) (pp. 77-82). IEEE.
Sachenko, B., Lysenko, S., Bobrovnikova, K., Savenko, O., &Markowsky, G. (2021, September). Detection DNS tunneling botnets. In 2021 11th IEEE International Conference on Intelligent Data Acquisition and Advanced Computing Systems: Technology and Applications (IDAACS) (Vol. 1, pp. 64-69). IEEE.
Ma D. J., Zhang S., Kong F., Fu Z.. (2021). MDN Detection Based on Doc2vec and Hybrid network. In IOP conference series: and Environmental Science. IOP publishing: Bristo, UK, Volume 693 [Cross Ref]
Palaniappan, G., Sangeetha, S., Rajendran, B., Goyal, S., &Bindhumadhava, B. S. (2020). Malicious domain detection using machine learning on domain name features, host-based features and web-based features. Procedia Computer Science, 171, 654-661.
Kwon J., Lee J., Lee H., and perring A. (2016). “PsyBog:Psy Bog: a scalable botnet detection method for large scale DNS Traffic”, Computer Networks vol.97, pp.48-73.
Woodbridge J., Anderson H.S., Ahuja A., Grant D. (2016). Predicting Domain Generation Algorithms with Long Short-Term Memory Networks.arXi,v arXiv:1611.00791.
Vinayakumar R., Soman K.P., Poornachandran P. (2018). Detecting MDNs using deep learning approaches at scale.J. Intell. Fuzzy System. 34, 1355-1367
Zang x., Gong j., and Hu X.. (2018). “Detecting MDN based on AGD”, Journal of International Technology, vol.39, no.7. pp. 15-25.
BharathiB.,andBhauvana J. (2019). Domain name detection and classification using deep neural networks. In international symposium on Security in Computing and Communication; Springer: Singapore.
Suliang Luo., Gang Han., An Li., JianlangPeng. (2022).“Detecting MDNs from domain generation algorithms using bi-directional LSTM network” Proc. SPIE 12455, International Conference on Signal Processing and Communication Security (ICSPCS) Dalian China. doi: 10.1117/12.2655176
Sachan, R. K., Agarwal, R., & Shukla, S. K. (2023). Identifying malicious accounts in blockchains using domain names and associated temporal properties. Blockchain: Research and Applications, 100136.
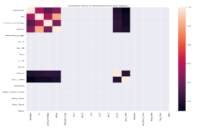
Downloads
Published
Issue
Section
License
Copyright (c) 2024 The Transactions of the Nigerian Association of Mathematical Physics

This work is licensed under a Creative Commons Attribution-NonCommercial 4.0 International License.