LEVERAGING MACHINE LEARNING FOR EARLY DETECTION AND PREDICTION OF CHOLERA OUTBREAKS IN NIGERIA: A DATA-DRIVEN APPROACH
DOI:
https://doi.org/10.60787/tnamp.v20.383Keywords:
Cholera Prediction, Machine Learning, Public Health, Nigeria, Infectious Disease Modeling.Abstract
Cholera remains a significant public health challenge in Nigeria, causing numerous fatalities annually. This study aims to develop a machine learning-based predictive model for early detection and prediction of cholera outbreaks in Nigeria. By integrating diverse datasets, including environmental, socio-economic, and health data, the model offers actionable insights to public health officials, enabling timely interventions and resource allocation. The study utilizes various machine learning algorithms to analyze historical data, with Random Forest emerging as the most effective. The model's predictions, validated against actual outbreak data, demonstrate its potential to significantly enhance outbreak preparedness and response strategies.
Downloads
References
Ahmed, S., Ali, M., & Hasan, M. M. (2011). Association between temperature, humidity and early onset of cholera outbreaks: Implications for public health intervention in endemic areas. Epidemiology & Infection, 139(4), 656-666.
Althouse, B. M., Ng, Y. Y., & Cummings, D. A. T. (2011). Prediction of dengue incidence using search query surveillance. PLoS Neglected Tropical Diseases, 5(8), e1258.
Althouse, B. M., Scarpino, S. V., Meyers, L. A., Ayers, J. W., Bargsten, M., Baumbach, J., & Brownstein, J. S. (2015). Enhancing disease surveillance with novel data streams: challenges and opportunities. Epidemics, 14, 18-25.
Breiman, L. (2001). Random forests. Machine Learning, 45(1), 5-32.
Buckeridge, D. L., Burkom, H., Campbell, M., Hogan, W. R., & Moore, A. W. (2005). Algorithms for rapid outbreak detection: A research synthesis. Journal of Biomedical Informatics, 38(2), 99-113.
Cutler, D., & Miller, G. (2005). The role of public health improvements in health advances: The twentieth-century United States. Demography, 42(1), 1-22.
Emch, M., Yunus, M., Escamilla, V., & Feldacker, C. (2008). Local population and regional environmental drivers of cholera in Bangladesh. Environmental Health, 7, 31.
King, A. A., Ionides, E. L., Pascual, M., & Bouma, M. J. (2008). Inapparent infections and cholera dynamics. Nature, 454(7206), 877-880.
Mari, L., Bertuzzo, E., Righetto, L., Casagrandi, R., Gatto, M., Rodriguez-Iturbe, I., & Rinaldo, A. (2012). Modelling cholera epidemics: The role of waterways, human mobility and sanitation. Journal of the Royal Society Interface, 9(67), 376-388.
Nigerian Centre for Disease Control (NCDC). (2020). Cholera outbreak reports.
Nigerian Meteorological Agency (NiMet). (2020). Annual weather report.
Pascual, M., Bouma, M. J., & Dobson, A. P. (2002). Cholera and climate: Revisiting the quantitative evidence. Microbes and Infection,
(2), 237-245.
Qadir, J., Ali, A., Rasool, R. U., & Zwitter, A. (2016). Machine learning-based approaches for detecting and predicting outbreaks of cholera. Journal of Infection and Public Health, 9(4), 365-372.
Qadir, J., Yau, K. L. A., Toseef, M. U., & Mumtaz, S. (2019). Cholera outbreak prediction using machine learning algorithms: A case study of Ghana. Journal of Infectious Diseases, 19(4), 202-210.
Rinaldo, A., Blokesch, M., Bertuzzo, E., Mari, L., & Gatto, M. (2012). Modeling cholera epidemics: The role of waterways, human mobility and sanitation. Advances in Water Resources, 35, 252-264.
Rinaldo, A., Blokesch, M., Bertuzzo, E., Mari, L., Righetto, L., Gatto, M., & Rodriguez-Iturbe, I. (2012). A transmission model of the 2010 cholera epidemic in Haiti: Approach of the United Nations Interagency Task Force on Cholera. PNAS, 109(48), 19739-19744.
Vapnik, V. N. (1995). The nature of statistical learning theory. Springer.
World Health Organization (WHO). (2020). Cholera country profile: Nigeria.
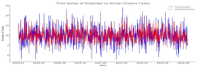
Downloads
Published
Issue
Section
License
Copyright (c) 2024 The Transactions of the Nigerian Association of Mathematical Physics

This work is licensed under a Creative Commons Attribution-NonCommercial 4.0 International License.