COMPARISON OF STATISTICAL MODEL AND RANDOM FOREST FOR GROUNDWATER CONTAMINATION PATTERNS.
DOI:
https://doi.org/10.60787/jnamp.v67i2.371Keywords:
Groundwater modelling, Contamination, Heavy Metals, Multiple Linear Regression, Random Forest ModelAbstract
In this study, a random forest model was compared to a statistical model for predicting heavy metal concentrations in groundwater in Edo State, Nigeria. The pH of groundwater samples was determined using a pH meter, and heavy metal concentrations were measured with Atomic Absorption Spectrophotometer (AAS). Pearson Correlation Coefficient was used to evaluate correlations between heavy metal concentrations. Both Random Forest Model (RFM) and Multiple Linear Regression (MLR) were employed to model these concentrations, with goodness of fit assessed via R-squared and root mean square error (RMSE). Results showed that heavy metal concentrations, except for lead, were generally within acceptable limits. The RFM outperformed MLR in predicting iron and lead concentrations but was less effective for arsenic. Python was used for modelling and data extraction. Both models are suitable for predicting groundwater contamination, with RFM showing better overall performance.
Downloads
References
Basu, A., Saha, D., Saha, R., Ghosh, T. and Saha, B. (2014). A review on sources, toxicity and remediation technologies for removing arsenic from drinking water. Res. Chem. Intermediation, 40:447–485.
Pandey, H.K., Duggal, S.K. and Jamatia, A. (2016). Fluoride contamination of groundwater and its hydrological evolution in District Sonbhadra (U.P.) India. Proc Nat Acad Sci India Sect A Phys Sci., 86:81–93.
Subba Rao, N., Ravindra B, Wu J (2020) Geochemical and health risk evaluation of fuoride rich groundwater in Sattenapalle Region, Guntur district, Andhra Pradesh, India. Hum Ecol Risk Assess, 26:2316–2348
He, X., Li, P., Ji, Y., Wang, Y., Su, Z. and Elumalai, V. (2020). Groundwater arsenic and fuoride and associated arsenicosis and fuorosis in China: occurrence, distribution and management. Expo Health, 12:355–368.
Li, P., Karunanidhi, D., Subramani, T. and Srinivasamoorthy, K. (2021). Sources and Consequences of Groundwater Contamination. Archives of Environmental Contamination and Toxicology (2021) 80:1–10.
Salem, M., Mohamed, E.L., Mossad, M. and Mahanna, H. (2022). Random forest modeling and evaluation of the performance of a full-scale subsurface constructed wetland plant in Egypt. Ain Shams Engineering Journal, 13(6): 101778.
Haggerty, R., Sun, J., Yu, H. and Li, Y. (2023). Application of machine learning in groundwater quality modeling - A comprehensive review, Water Research, 233: 119745.
Cracknell MJ (2014) Machine learning for geological mapping: algorithms and applications. Ph.D. University of Tasmania, Tasmania.
Madani, A., Hagage, M. and Elbeih, S.F. (2022). Random Forest and Logistic Regression algorithms for prediction of groundwater contamination using ammonia concentration.Arabian Journal of Geosciences, 15(1619): 1 - 12.
Gislason PO, Benediktsson JA, Sveinsson JR (2006) Random forests for land cover classifcation. Pattern Recognit. Lett., 27 (4):294–300.
Pham BT, Prakash I (2019) A novel hybrid model of Bagging-based Naïve Bayes Trees for landslide susceptibility assessment. Bull. Eng. Geol. Environ., 78(3):1911–1925.
Ouedraogo, I., Defourny, P. and Vanclooster, M. (2018). Application of random forest regression and comparison of its performance to multiple linear regression in modeling groundwater nitrate concentration at the African continent scale. Hydrogeology Journal, 4(1), 23 - 29.
Cho, K.H., Sthipong, S., Pachepsky, Y.A., Kyoung-Woong, K. and Kim, J.H. (2011). Prediction of contamination potential of groundwater arsenic in Cambodia, Laos, and Thailand using artificial neural network. Water research, 45(17): 5535-5544.
WHO (2017). Guidelines for drinking-water quality, 4th edition, incorporating the 1st addendum. World Health Organization.
NIS (2015). Nigerian Standard for Drinking Water Quality. Federal Ministry of Water Resources, Nigeria.
Cirino, E. (2019). What pH Should My Drinking Water Be? Healthline Media LLC. pH of Drinking Water: Acceptable Levels and More (healthline.com).
Omonona, A.O., Solotan, T.V. and Adetuga, A.T. (2020). Heavy Metals Contamination in Water, Soil and Plant Samples of Okomu National Park, Edo State, Nigeria. Afr. J. Biomed. Res., 23: 29 - 34.
Odiana, S. and Edosomwan, E.U. (2019). Heavy metal concentrations in domestic water sources in some communities of Edo state, Nigeria. FUW Trends in Science & Technology Journal, 4(2): 539 - 544.
Wei, H., Yu, H., Zhang, G., Pan, H., Lvb, C. and Meng, F. (2018). Revealing the correlations between heavy metals and water quality, with insight into the potential factors and variations through canonical correlation analysis in an upstream tributary. Ecological Indicators, 90: 485–493.
Canli, M. and Atli, G. (2003). The relationships between heavy metal (Cd, Cr, Cu, FE, Pb, Zn) levels and the size of six Mediterranean fish species. Environ. Pollut. 121 (1): 129–136.
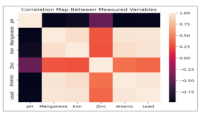
Downloads
Published
Issue
Section
License
Copyright (c) 2024 The Journals of the Nigerian Association of Mathematical Physics

This work is licensed under a Creative Commons Attribution-NonCommercial-ShareAlike 4.0 International License.