NEURAL NETWORK MODELING OF RELATIVE HUMIDITY AND TEMPERATURE DISTRIBUTION OVER NIGERIA
Keywords:
Nigeria, Adiabatic, Diabatic, Neural Network, Temperature, Relative HumidityAbstract
This paper used neural network model to study the distributions of relative humidity and temperature over Nigeria. The theoretical explorations of the relationship between the parameters were reviewed. This study was carried out on thirty-six point stations
over Nigeria. Temporal variations of estimation and prediction of relative humidity and temperature were carried out in this study. The results revealed that temperature and relative humidity distributions over Nigeria are in variant. They were inversely proportional to each other as affirmed by other researched. Spatio-temporal variations revealed that relative humidity is higher in wet seasons compared to dry seasons in Nigeria. It is also higher within the Southern part of Nigeria as a result of coastal nature, moisture content in the atmosphere of the region and low temperature gradient. The influences of temperature on relative humidity were study. The results shows inversion with the rates of relative humidity and the rates of temperature both in wet and dry seasons, and within the Southern and Northern part of Nigeria. This could be because increase in temperature raises saturated vapour pressure, which leads to reduction in the relative humidity. The variation of temperature may be due to diabatic heating and adiabatic effects in the atmosphere. Based on the similar signatures of the estimated and observed temporal distributions of the parameters, forecast of two years ahead of the years of the study were successfully achieved. The result showed strong negative relationship between temperature and relative humidity. The correlation coefficient is calculated to be -0.94. This strong negative correlation signifies that as the temperature decreases, the relative humidity increase (and vice versa). The performance of neural network model in the distributions shows the ability of the model in studying atmospheric parameters as confirmed by other researchers.
Downloads
References
Xin L, Wenyou F, Lunche W, Ming L, Rui Y, Shaoqiang W, Lizhe W (2020) Effect of urban expansion on atmospheric humidity in Beijing-Tianjin-Hebei urban agglomeration. Sci of the Tot Env 759: 1-2.
Lawrence MG (2005) The Relationship between Relative Humidity and the Dewpoint Temperature in Moist Air: A Simple Conversion and Applications. Am Met So 86 (2): 225-233
Ibeh GF, Agbo GA, Agbo PE, Ali PA (2012) Application of Neural Network for Global Solar Radiation Forecasting with Temperature. Adv in Appl Sci Res 3(1):130-134.
Ibeh, Ibeh, Ogbonna, Ofoma and Akande J. of NAMP Heyn I, Quaas J, Salzmann M, Mülmenstädt J (2017) Effects of Diabatic and Adiabatic processes on Relative Humidity in a GCM, and Relationship between Mid-Tropospheric Vertical Wind and Cloud- Forming and Cloud-Dissipating Processes. Dyn Met and Ocea 69:1, 1-14. DOI: 0.1080/16000870.2016.1272753
Kinza Q, Ashfaq A, Muhammad A, Abdul-Sattar N, Moonyong L (2021) Developing Machine Learning Models for Relative Humidity Prediction in Air-Based Energy Systems and Environmental Management Applications. J of Env Mgt 292:1-10. DOI: 10.1016/j.jenvman.2021.112736
Buhari M and Adamu SS (2012) Short-Term Load Forecasting Using Artificial Neural Network. Proceedings of international Multi-Conference of Engineers and Computer Scientists. Vol 1 IMECS Hong Kong 2-14
Kevin PM (2012) Machine Learning: A Probabilistic Perspective. The MIT Press Cambridge, Massachusetts, London, England. 1-2
Annina S, Deo MS, Venkatesan S, Ramesh BDR (2015). An overview of Machine Learning and its Applications. Inter J of Elect Sci and Eng(IJESE) 1 (1): 22-24.
Alzubi J, Nayyar A, Kumar A (2018) Machine Learning from Theory to Algorithms: An Overview. J of Phy: Conf. Series 1142: 1-15
Agbulut Ü, Gürel AE, Ergün A, Ceylan˙I (2020) Performance assessment of a V-trough photovoltaic system and prediction of power output with different machine learning algorithms. J. Clean. Prod 268: 1-7. https://doi.org/10.1016/j. jclepro.2020.122269.
Assouline D, Mohajeri N, Scartezzini J (2018) Large-scale rooftop solar photovoltaic technical otential estimation using Random Forests. Appl.
Energy 217: 189–211. https://doi.org/10.1016/J.APENERGY.2018.02.118.
Li Y, Zou C, Berecibar M, Nanini-Maury E, Chan JCW, Van DBP, Mierlo J, Omar N (2018) Random forest regression for online capacity estimation of lithium-ion batteries. Appl. Energy 232: 197–210. https://doi.org/ 10.1016/j.apenergy.2018.09.182.
Yang Q, Yuan Q, Li T, Yue L (2020) Mapping PM2.5 concentration at high resolution using a cascade random forest based downscaling model: evaluation and application. J. Clean. Prod 277: 1-7. https://doi.org/10.1016/j.jclepro.2020.123887
Beale MH, Haagan MT, Demuth BH (2014) Neural Network ToolboxTM. User’s Guide (R2014b) USA, The Math Works, Incorporation Pp 10-40
Sheela KG, Deepa SN (2013) A new algorithm to find number of hidden neurons in Radia Basis Function Networks for wind speed prediction in renewable energy systems. Con Eng Appl Inf 15(3): 30-37.
Daniel O, Najib Y, Oluwaseye A, Ibrahim M, Bababtunde R (2015) Preliminary results of temperature modeling in Nigeria using neural networks. Roy Met Soci (70): 336-342.
Maitha HSA, Ali HA, Hassan ANH (2011) Using MATLAB to Artificial Neural Network Models for Predicting Global Solar Radiation in Al Ain City–UAE Using MATLAB code. Engineering Education and Research Using MATLAB 9: 220-238
Ibeh G. F, Udochukwu B. C, Ibeh L. M and Okoh D (2020). Evaluation of methane emission distributions in Nigeria using neural network model. Climate Change - Disc Scie Soc, 6 (21).
Ibeh GF, Udochukwu BC, Igbawua T, Tyovenda A, Ofoma JN (2019) Assessment of Global Solar Radiation at Selected Points in Nigeria Using Artificial Neural Network Model (ANNM). Inter J of Env and Clim Ch 9(7): 376-390
Eludoyin OM (2011) Air Temperature and Relative Humidity Areal Distribution over Nigeria. IFE Res Pub in Geo 10(1): 134 – 145
Ajadi DA, Sanusi YK (2013) Effect of Relative Humidity on Oven Temperature of Locally Design Solar Carbinet Dryer. Glob J of Sci Front Res Phy and Spa Sci 13 (1): 13-17
Ibeh, Ibeh, Ogbonna, Ofoma and Akande J. of NAMP Mawonike R, Mandonga G (2017). The Effect of Temperature and Relative Humidity on Rainfall in Gokwe Region, Zimbabwe: A Factorial Design Perspective. Inter J of Mult Aca Res 5 (2): 2309-3218
Jirasuwankul N (2018) Visualization and Estimation of Temperature from Glowing Hot Object by Artificial Neural Network and Image Analysis Technique. The 8th International Conference on SustainableEnergy Information Technology (SEIT 2018), Sci Dir Procedia Comp Sci 130: 712–719
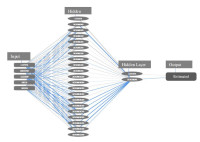
Downloads
Published
Issue
Section
License
Copyright (c) 2024 The Journals of the Nigerian Association of Mathematical Physics

This work is licensed under a Creative Commons Attribution-NonCommercial-ShareAlike 4.0 International License.