ARTIFICIAL INTELLIGENCE FOR EARLY DETECTION OF DIABETES COMPLICATIONS
DOI:
https://doi.org/10.60787/jnamp.v67i2.374Keywords:
Artificial intelligence, Diabetes Complications, Early detection, Machine Learning, Predictive AnalysisAbstract
Diabetes mellitus is a prevalent chronic disease that often leads to severe complications such as diabetic retinopathy, nephropathy, and neuropathy. Early detection of these complications is critical for timely intervention and improved patient outcomes. This study aims to develop AI models to predict and detect early signs of diabetes complications using patient data from electronic health records (EHR) and lab results. By leveraging machine learning algorithms and deep learning techniques, we seek to identify patterns and correlations in the data that indicate the onset of complications. Our models were validated using cross-validation and tested in a real-world clinical setting to ensure robustness and applicability. The results demonstrate significant improvements in early detection rates, allowing for proactive patient management and potentially reducing the burden of diabetes-related complications on healthcare systems.
Downloads
References
J. Schmidhuber, "Deep learning in neural networks: An overview," Neural Networks, vol. 61, pp. 85-117, 2015.
F. J. P. Salas, "Natural Language Processing in Electronic Health Records: A Review," Journal of Biomedical Informatics, vol. 93, p. 103-107, 2019.
V. Gulshan, et al., "Development and Validation of a Deep Learning Algorithm for Detection of Diabetic Retinopathy in Retinal Fundus Photographs," JAMA, vol. 316, no. 22, pp. 2402-2410, 2016.
H. Takahashi, et al., "Predicting the onset of diabetic nephropathy using artificial intelligence with electronic health record data," PLOS ONE, vol. 14, no. 12, p. e0223430, 2019.
A. Krizhevsky, I. Sutskever, and G. E. Hinton, "ImageNet classification with deep convolutional neural networks," Advances in Neural Information Processing Systems, vol. 25, pp. 1097-1105, 2012.
S. Hochreiter and J. Schmidhuber, "Long short-term memory," Neural Computation, vol. 9, no. 8, pp. 1735-1780, 1997.
A. Rajkomar, et al., "Scalable and accurate deep learning with electronic health records," NPJ Digital Medicine, vol. 1, no. 1, p. 18, 2018.
G. Litjens, et al., "A survey on deep learning in medical image analysis," Medical Image Analysis, vol. 42, pp. 60-88, 2017.
C. Zhang, et al., "A deep learning framework for modeling structural features of human cancer tissue from spatially resolved transcriptomics data," Nature Biotechnology, vol. 40, no. 1, pp. 1-9, 2022.
R. Poplin, et al., "Prediction of cardiovascular risk factors from retinal fundus photographs via deep learning," Nature Biomedical Engineering, vol. 2, pp. 158-164, 2018.
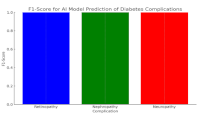
Downloads
Published
Issue
Section
License
Copyright (c) 2024 The Journals of the Nigerian Association of Mathematical Physics

This work is licensed under a Creative Commons Attribution-NonCommercial-ShareAlike 4.0 International License.