Application Of Mathematical Convolution Approach Of Image Sharpening To Digital And Satellite Imaging
DOI:
https://doi.org/10.60787/jnamp.v68no1.412Keywords:
Mathematical convolution, Image sharpening, Laplacian, Digital imaging, KernelAbstract
This research paper explores the roles of convolution and Laplacian operators in image sharpening, with the primary objective of enhancing image clarity. The study delves into the mathematical foundations of these operators and their
application in digital image processing. Methods are compared to evaluate their effectiveness in terms of computational efficiency and visual outcomes. The paper identifies the gaps in existing literature, particularly the lack of comprehensive literature on the derivation of the Laplacian kernel and reasons for post-processing methods after its convolution with an image. The literature in this paper helps understand the foundation of Laplacian kernel, offering valuable insights on its derivation, and setting the basis for other techniques that have been built on it, with
applications in satellite imagery and digital photography. Also, comparison of Laplacian kernel with unsharp masking method is presented.
Downloads
References
Morteza Ghahremani and Hassan Ghassemian. “Remote sensing image fusion using ripplet transform and compressed sensing”. In: IEEE Geoscience and Remote Sensing Letters 12.3 (2014), pp. 502–506.
Dhara J. Sangani, Rajesh A. Thakker, S.D. Panchal, and Rajesh Gogineni. “Pansharpening of satellite images with convolutional sparse coding and adaptive PCNN-based approach”. In: Journal of the Indian Society of Remote Sensing 49.12 (2021), pp. 2989–3004.
Rafael C. Gonzalez and Richard E. Woods. Digital Image Processing. 3rd ed. Pearson Prentice Hall, 2008.
Wenming Tang, Yuanhao Gong, Linyu Su, Wenhui Wu, and Guoping Qiu. “Structure adaptive filtering for edge-preserving image smoothing”. In: Image and Graphics: 11th International Conference, ICIG 2021, Haikou, China, August 6–8, 2021, Proceedings, Part III 11 (2021), pp. 265–276.
Hui Lv, Pengfei Shan, Hongfang Shi, and Li Zhao. “An adaptive bilateral filtering method based on improved convolution kernel used for infrared image enhancement”. In: Signal, Image and Video Processing 16.8 (2022), pp. 2231–2237.
Li-bao Zhang, Yang Sun, and Jue Zhang. “Pan-sharpening based on common saliency feature analysis and multiscale spatial information extraction for multiple remote sensing images”. In: International Journal of Remote Sensing 41 (2020), pp. 3095–3118.
Honglyun Park, Namkyung Kim, Sangwoo Park, and Jaewan Choi. “Sharpening of Worldview-3 Satellite Images by Generating Optimal High-Spatial-Resolution Images”. In: Applied Sciences (2020). doi: 10.3390/app10207313.
Kholmat Shadimetov and Shermamat Esanov. “The discrete convolution operator D m [????]”. In: AIP Conference Proceedings. Vol. 2365. 1. AIP Publishing LLC. 2021, p. 020033.
Giulio Prevedello and Ken R Duffy. “Discrete convolution statistic for hypothesis testing”. In: Communications in Statistics-Theory and Methods 51.12 (2022), pp. 4097–4118.
Richard Szeliski. Computer vision: algorithms and applications. Springer Nature, 2022. 18
William K Pratt. Digital image processing: PIKS Scientific inside. Vol. 4. Wiley Online Library, 2007.
Thomas R Edwards. “Two-dimensional convolute integer operators for digital image processing”. In: Image Processing Algorithms and Techniques. Vol. 1244. SPIE. 1990, pp. 26–44.
Gengxiang Chen et al. “Laplace neural operator for complex geometries”. In: arXiv preprint arXiv:2302.08166 (2023).
Robert Dautray and Jacques-Louis Lions. “The Laplace Operator”. In: Mathematical Analysis and Numerical Methods for Science and Technology. Springer, 2000, pp. 220– 658.
John C Russ. The image processing handbook. CRC press, 2006.
Raman Maini and Himanshu Aggarwal. “Study and comparison of various image edge detection techniques”. In: International journal of image processing (IJIP) 3.1 (2009), pp. 1–11.
Maria MP Petrou and Costas Petrou. Image processing: the fundamentals. John Wiley & Sons, 2010.
Haotian Xu, Weiwei Xu, and Wenchao Chen. “Seismic erratic noise suppression based on Laplacian-scaled mixture prior”. In: Second International Meeting for Applied Geoscience & Energy. Society of Exploration Geophysicists and American Association of Petroleum . . . 2022, pp. 2967–2971.
Xiucai Ding and Hau-Tieng Wu. “Impact of signal-to-noise ratio and bandwidth on graph Laplacian spectrum from high-dimensional noisy point cloud”. In: IEEE Transactions on Information Theory 69.3 (2022), pp. 1899–1931.
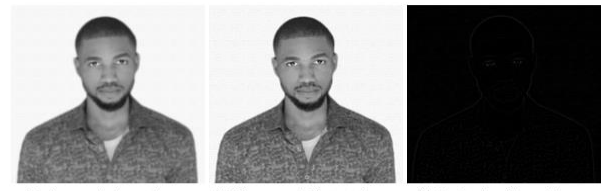
Downloads
Published
Issue
Section
License
Copyright (c) 2024 The Journals of the Nigerian Association of Mathematical Physics

This work is licensed under a Creative Commons Attribution-NonCommercial-ShareAlike 4.0 International License.