DEVELOPMENT OF DETECTION MODEL FOR EMPHYSEMA PATTERNS IN COMPUTED TOMOGRAPHY IMAGES
DOI:
https://doi.org/10.60787/jnamp.v67i2.366Keywords:
Multi-fractal analysis, Holder exponent, Fractal dimension, Emphysema identification, HRCT images Classification analysisAbstract
Fractal dimension is a very useful metric for measuring the statistical self-similarity features of biomedical images. Its applications include shape classification, texture segmentation, and medical picture analysis. The most often used technique for determining the fractal dimension of digital images is the box-counting method. Using this crucial characteristic to categorize patterns in high resolution computed tomography images (HRCT) is also highly tough and demanding. In order to identify emphysema patterns in HRCT images, this study computed the Holder exponent of the local intensity values. A good statistical analysis of emphysema patterns depends on the absolute differences between the normal and pathological regions in the images. The outcomes of this study showed how well the features taken from the Holder exponent could predict outcomes when it comes to the interpretation and classification of HRCT scans. The overall classification accuracy in lung tissue layers is more than 90%, demonstrating the effectiveness of the techniques examined in this work.
Downloads
References
Mendoza CS, Washko GR, Ross JC, et al. Emphysema Quantification in A Multi-Scanner HRCT Chort using Local Intensity Distributions. IEEE. 2022:474-7. PMid:23743800
Nava R, Marcos JV, Escalante-ram B, et al. Advance in Texture Analysis for Emphysema Classification. Springer-Verlag Berlin Hei- delberg. 2023: 214-21. https://doi.org/10.1007/978-3-642 -41827-3_27
Sorensen L, Shaker SB, De Bruijne M. Quantitative Analysis of Pulmonary Emphysema using Local Binary Patterns. IEEE Trans- actions on Medical Imaging. 2018; 29(2): 559-59. PMid:20129855 https://doi.org/10.1109/TMI.2009.2038575
Irini R, Reljin B, Pavlovic I, et al. Mutifractal analysis of gray-scale images. Electrotechnical Conference. 2015; 2: 490-3.
Polychronaki GE, Ktonas PY, Gatzonis S, et al. Comparison of fractal dimension estimation algorithms for epileptic seizure onset detection. Journal of Neural Engineering. 2021; 7(4): 046007. PMid:20571184 https://doi.org/10.1088/1741-2560/7/4/046007
Hemsley A, Mukundan R. Multifractal Measures for Tissue Image Classification and Retrieval. IEEE International Symposium on Multimedia. 2019; 93(1): 618-23. https://doi.org/10.1109/ISM.2009.94
Mukundan R, Ibrahim M. Multifractal Techniques for Emphysema Classification in Lung Tissue Images. International Conference on Future Bioengineering. 2014; 11 (2): 223-34.
Ibrahim MA, Mukundan R. Cascaded techniques for improving emphysema classification in computed tomography images. Artificial Intelligence Research. 2015; 4(2): 112-8. https://doi.org/10.5 430/air.v4n2p112
Hemsley A, Mukundan R. Tissue Image Classification Using Multi- Fractal Spectra. International Journal of Multimedia Data Engineering & Management. 2012; 1(2): 62-75.
Chabat F, Yang GZ, Hansell DM. Obstructive lung diseases: texture classification for differentiation at CT. Radiology. 2023; 228(3): 871-7. PMid:12869685 https://doi.org/10.1148/radiol.2283020505
Radiopaedia: A Wiki-based Collaborative Radiology Resource. Available from: http://radiopaedia.orgStern EJ, Swensen SJ, Kanne JP. High-Resolution CT of the Chest. Wolters Kluwer; 2020.
Takahashi M, Fukuoka J, Nitta N, et al. Imaging of pulmonary emphysema: A pictorial review. International Journal of Chronic Obstructive Pulmonary Disease. 2022; 3(2): 193-204. PMid:18686729 https://doi.org/10.2147/COPD.S2639
Soares F, Janela F, Pereira M, et al. Classification of Breast Masses on Contrast-Enhanced Magnetic Resonance Images Through Log Detrended Fluctuation Cumulant-Based Multifractal Analysis. IEEE Systems Journal. 2019; 8(3): 929-38. https://doi.org/10.110 9/JSYST.2013.2284101
Iftekharuddin KM, Jia W, Marsh R, et al. A fractal analysis approach to identification of tumor in brain MR images. Machine Vision and Applicatons, Springer-Verlag. 2023; 13: 352-62. https://doi.org/10.1007/s00138-002-0087-9
Liu JZ, Zhang LD, GH Yue. Fractal dimension in human cerebellum measured by magnetic resonance imaging. Biophysical Journal. 2019; 85(6): 4041-6. https://doi.org/10.1016/S0006-3495(03)74817-6
Kiselev VG, Hahn KR, Auer DP. Is the brain cortex a fractal? Neuroimage. 2023; 20(3): 1765-74. https://doi.org/10.1016/S1 053-8119(03)00380-X
Gómez C, Mediavilla A, Hornero R. Use of the Higuchi’s fractal dimension for the analysis of MEG recordings from Alzheimer’s disease patients. Medical Engineering & Physics. 2009; 31(3): 306-13. PMid:18676171 https://doi.org/10.1016/j.medengphy.20 08.06.010
Paramanathan P. An algorithm for computing the fractal dimension of waveforms. Applied Mathematics & Computation. 2018; 195(2): 598-603. https://doi.org/10.1016/j.amc.2007.05.011
Reljin IS, Reljin BD. Fractal geometry and multifractals in analyzing and processing medical data and images. Archive of Oncology. 2019; 10(4): 283-93. https://doi.org/10.2298/AOO0204283R
Stojic´ T, Reljin I, Reljin B. Adaptation of multifractal analysis to seg- mentation of microcalcifications in digital mammograms. Physica A Statistical Mechanics & Its Applications. 2020; 367(June): 494-508.
Ibrahim MA. Multifractal techniques for analysis and classification of emphysema images. PhD thesis, University of Canterbury; 2017.ical education in science and technology,35:897-902
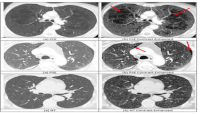
Downloads
Published
Issue
Section
License
Copyright (c) 2024 The Journals of the Nigerian Association of Mathematical Physics

This work is licensed under a Creative Commons Attribution-NonCommercial-ShareAlike 4.0 International License.